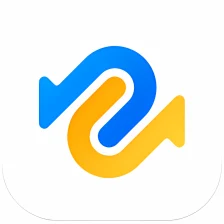
Tenorshare 4DDiG Data Recovery (Activation number included)
Last Updated:20-07-2024, 09:47
dataredning
About
dataredning
Understanding Data Cleaning (Dataredning) Understanding Data Cleaning (Dataredning)Data cleaning, or 'dataredning' in Swedish, is a critical process in data management that involves identifying and correcting or removing inaccuracies, inconsistencies, and irrelevant parts of the data set. This article delves into the intricacies of data cleaning, its importance, methods, tools, and best practices.
Introduction to Data Cleaning (Dataredning)
Data cleaning is an essential step in the data analysis process. It ensures that the data used for analysis is accurate, complete, and consistent. Poorly cleaned data can lead to incorrect conclusions and flawed business decisions. This section introduces the concept of data cleaning, its definition, and its significance in data-driven decision-making.
Definition of Data Cleaning (Dataredning)
Data cleaning, or dataredning, refers to the process of detecting and correcting (or removing) corrupt or inaccurate records from a record set, table, or database. It involves identifying incomplete, incorrect, inaccurate, or irrelevant parts of the data and then replacing, modifying, or deleting this bad data.
Importance of Data Cleaning (Dataredning)
The importance of data cleaning cannot be overstated. Clean data is crucial for reliable analysis and decision-making. It enhances the quality of data, improves the accuracy of models, and ensures that insights drawn from the data are valid and actionable. This subsection explores why data cleaning is vital for organizations across various industries.
Methods of Data Cleaning (Dataredning)
There are several methods and techniques used in data cleaning. These methods are designed to address different types of data issues such as missing values, duplicate entries, inconsistent formats, and outliers. This section discusses various data cleaning methods and how they are applied in practice.
Handling Missing Values
Missing data is a common issue in datasets. It can be handled in several ways, including deletion of rows with missing values, imputation using statistical methods, or using machine learning algorithms to predict missing values. This subsection details the strategies for managing missing data effectively.
Dealing with Duplicates
Duplicate data can skew analysis results and lead to incorrect conclusions. Identifying and removing duplicates is a crucial part of data cleaning. This subsection explains how to detect and eliminate duplicate entries in a dataset.
Standardizing Data Formats
Inconsistent data formats can complicate data analysis. Standardizing formats such as dates, addresses, and categorical variables is essential for maintaining data consistency. This subsection provides insights into how to standardize data formats during the cleaning process.
Outlier Detection and Treatment
Outliers are data points that are significantly different from others and can affect the accuracy of statistical analyses. Detecting and appropriately treating outliers is a key aspect of data cleaning. This subsection discusses methods for identifying and handling outliers.
Tools for Data Cleaning (Dataredning)
Several tools and software are available to assist with data cleaning. These tools automate many of the tasks involved in data cleaning, making the process more efficient and less error-prone. This section reviews some of the most popular data cleaning tools and their features.
OpenRefine
OpenRefine is a powerful tool for working with messy data. It offers features like data transformation, clustering, and faceted browsing. This subsection explores how OpenRefine can be used for effective data cleaning.
Trifacta Wrangler
Trifacta Wrangler is a user-friendly tool that helps in cleaning and transforming raw data into structured formats. It uses machine learning to assist users in cleaning their data. This subsection details the capabilities and benefits of using Trifacta Wrangler.
DataCleaner
DataCleaner is an open-source data profiling and data quality analysis tool. It helps in identifying data quality issues and provides solutions for cleaning the data. This subsection discusses the features and applications of DataCleaner.
Best Practices in Data Cleaning (Dataredning)
Implementing best practices in data cleaning can significantly improve the quality of data and the efficiency of the cleaning process. This section outlines some of the best practices that data professionals should follow to ensure effective data cleaning.
Establishing Clear Data Cleaning Standards
Having clear standards for data cleaning ensures consistency and quality across different datasets. This subsection discusses how to establish and implement data cleaning standards within an organization.
Regular Data Audits
Regular audits of data can help in identifying and addressing data quality issues proactively. This subsection explores the benefits of conducting regular data audits and how to implement them.
Using Automated Tools
Automated tools can significantly reduce the time and effort required for data cleaning. This subsection provides insights into how organizations can leverage automated tools to enhance their data cleaning processes.
Training and Skill Development
Training staff in data cleaning techniques and tools is essential for maintaining high-quality data. This subsection discusses the importance of training and skill development in data cleaning.
Conclusion
Data cleaning, or dataredning, is a fundamental process that ensures the accuracy and reliability of data used for analysis and decision-making. By understanding the methods, tools, and best practices of data cleaning, organizations can enhance the quality of their data and make more informed decisions. This article has provided a comprehensive overview of data cleaning, highlighting its importance and the various aspects involved in the process.